AI used to predict which animal viruses are likely to infect humans: study
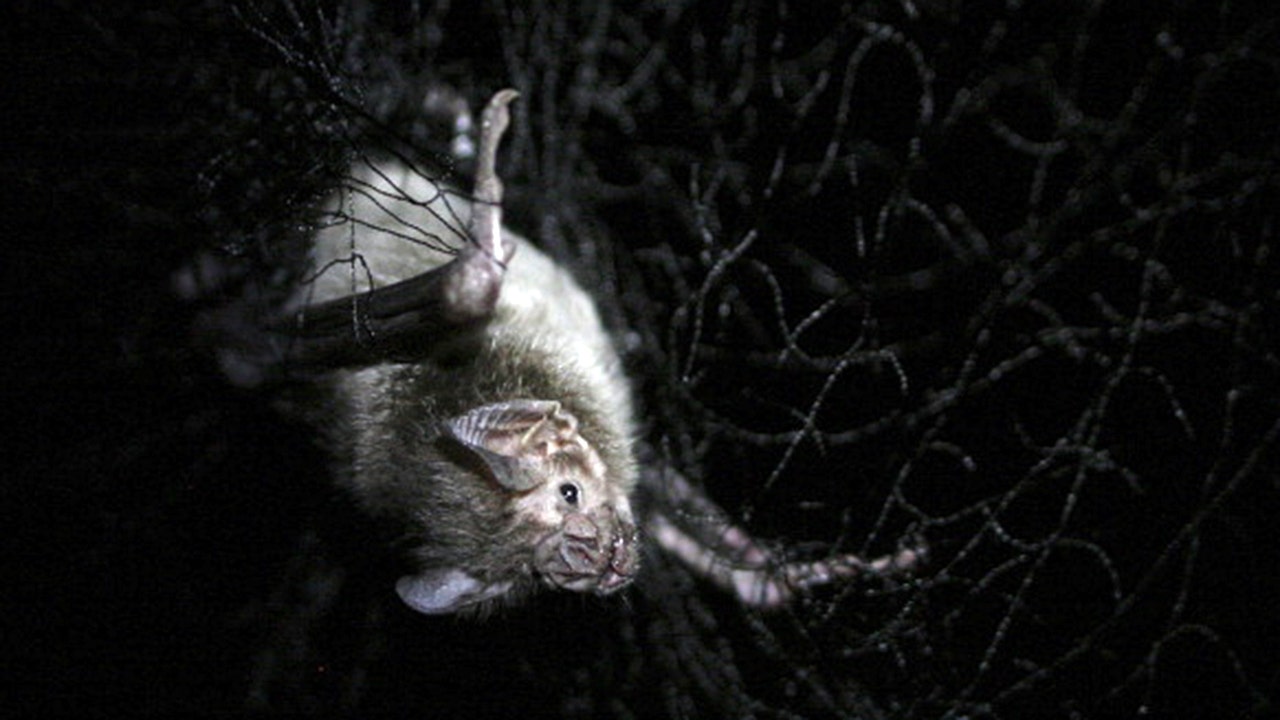
Artificial intelligence (AI) could be key in helping scientists identify the next animal virus that is capable of infecting humans, according to researchers.
In a Tuesday study published in the journal PLoS Biology, the Glasgow-based team said it had devised a genomic model that could “retrospectively or prospectively predict the probability that viruses will be able to infect humans.”
The group developed machine learning models to single out candidate zoonotic viruses using signatures of host range encoded in viral genomes.
With a dataset of 861 viral species with known zoonotic status, the researchers collected a single representative genome sequence from the hundreds of RNA and DNA virus species, spanning 36 viral families.
They classified each virus as being capable of infecting humans or not, made by merging three previously published datasets that reported data at the virus species level and did not consider the potential for variation in host range within virus species.
The researchers trained models to classify viruses accordingly.
Binary predictions correctly identified nearly 72% of the viruses that predominantly or exclusively infect humans and nearly 70% of zoonotic viruses as human infecting, though performance varied among viral families.
Upon further conversion of predicted probabilities of zoonotic potential into four categories, 92% of human-infecting viruses were predicted to have medium, high or very high zoonotic potential and a total of 18 viruses not currently considered to infect humans by their criteria were projected to have very high zoonotic potential – at least three of which had serological evidence of human infection, suggesting they could be valid zoonoses.
“Across the full dataset, 77.2% of viruses predicted to have very high zoonotic potential were known to infect humans,” the researchers wrote.
Next, the scientists tested several learning-based models to find the best-performing model, which was used to rank 758 virus species – and 38 viral families – not present in training data.
Among a second set of 645 animal-associated viruses excluding from training data, models predicted increased zoonotic transmission risk of genetically similar nonhuman primate-associated viruses.
YOUR APPS ARE WATCHING EVERYWHERE YOU GO UNLESS YOU CHANGE THIS SETTING
“Taken together, our results are consistent with the expectation that the relatively close phylogenetic proximity of nonhuman primates may facilitate virus sharing with humans and suggest that this may in part reflect common selective pressures on viral genome composition in both humans and nonhuman primates. However, broad differences among other animal groups appear to have less influence on zoonotic potential than virus characteristics,” the authors said.
In total, 70.8% of viruses sampled from humans were correctly identified with high or very high zoonotic potential.
A second case study predicted the zoonotic potential of all currently recognized coronavirus species and the human and animal genomes of all severe acute respiratory syndrome-related coronavirus.
“Our findings show that the zoonotic potential of viruses can be inferred to a surprisingly large extent from their genome sequence,” the researchers reported. “By highlighting viruses with the greatest potential to become zoonotic, genome-based ranking allows further ecological and virological characterization to be targeted more effectively.”
By identifying high-risk viruses and conducting further investigation, they said predictions could aid in the growing imbalance between the rapid pace of virus discovery and research needed to comprehensively evaluate risk.
Nearly 2 million animal viruses can infect humans.
“Importantly, given diagnostic limitations and the likelihood that not all viruses capable of human infection have had opportunities to emerge and be detected, viruses not reported to infect humans may represent unrealized, undocumented, or genuinely nonzoonotic species. Identifying potential or undocumented zoonoses within our data was an a priori goal of our analysis,” the group said.
CLICK HERE TO GET THE FOX NEWS APP
“A genomic sequence is typically the first, and often only, information we have on newly discovered viruses, and the more information we can extract from it, the sooner we might identify the virus’ origins and the zoonotic risk it may pose,” co-author Simon Babayan of the Institute of Biodiversity at the University of Glasgow said in a journal news release.
“As more viruses are characterized, the more effective our machine learning models will become at identifying the rare viruses that ought to be closely monitored and prioritized for preemptive vaccine development,” he added.